Main Track: Environmental Monitoring
- 11.10.23
- 13:30 - 15:00
- Chair : Kostas Karazas
Towards Fine-Grained Sensor-Based Probabilistic Individual Air Pollution Exposure Prediction using Wind Information
- Till Riedel
- KIT
- Sylvenstein
- 13:30
The estimation of pollutant exposure is highly dependent on the spatial and temporal resolution of the underlying model. This work presents a street-level Gaussian Process Regression model for urban air quality that uses a novel covariance kernel based on physical considerations to process wind information. This model can be driven by information from observations from low-cost sensor networks. We present the model, including the construction of the wind angle kernel, and discuss the inconclusive evaluation to date, the current challenges, and the way forward.
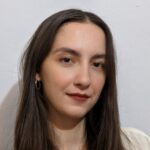
everWeather: A Low-Cost and Self-Powered AIoT Weather Forecasting Station for Remote Areas
- Sofia Polymeni
- Information Technologies Institute, Centre for Research and Technology Hellas
- Sylvenstein
- 14:00
Weather constitutes a crucial factor that impacts many of the human outdoor activities, whether they are related to obligations or pleasure. In the contemporary era, due to climate change, the weather is more unstable and the forecasting task is more challenging than ever. By combining the Internet of Things (IoT) with Artificial Intelligence (AI), a new research field emerges that is called Artificial Intelligence of Things (AIoT) and could offer significant possibilities for the research community in order to efficiently tackle the short-term weather forecasting. Renewable energy sources constitute solutions for the achievement of sustainability development goals and could also offer power autonomy in a weather forecasting station. In the present research study, everWeather is proposed as a low-cost, self-powered weather forecasting station based on the AIoT paradigm and renewable energy. The proposed solution combines a variety of low-cost environmental sensors, the prowess of solar energy and an appropriate lightweight Machine Learning (ML) algorithm such as the Multiple Linear Regression (MLR) in order to forecast physical weather for the next half hour. Preliminary experiments have been conducted for the proposed solution validation and the corresponding results highlighted that the performance of the everWeather station is quite satisfactory, in terms of reliability and forecasting accuracy.
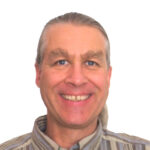
Management of climate protection measures in peatland areas in Schleswig-Holstein
- Friedhelm Hosenfeld
- DigSyLand
- Sylvenstein
- 14:30
The peatland database application Schleswig-Holstein is presented, a web application for the management of geospatial and non-geospatial data covering peatland protection, which was designed and implemented on behalf of the nature conservation departments of the Ministry for Energy Transition, Climate Protection, Environment and Nature of Schleswig-Holstein (MEKUN) and the State Agency for the Environment of Schleswig-Holstein (LfU). The development of the web application was based on the IT requirements for the collection, research, evaluation, and reporting of peatland data. Other applications already used by the nature conservation authorities, such as a nature conservation measures database and a protected areas register, were linked to the peatland database. The implementation was carried out as a PHP application that was integrated into the Disy Cadenza evaluation and GIS platform. Particular challenges included dynamic integration of measures from the nature conservation measures database. For restoration measures, a function is provided that automatically determines the reduction of CO2 emissions.